From Visibility to Control - Key Takeaways from a Fireside Chat with Viktor Farcic & Shmuel Kliger
“You actually cannot do meaningful reasoning especially when it comes to root cause analysis with LLMs or machine learning alone. You need more than that.” -Shmuel Kliger, Founder of Causely
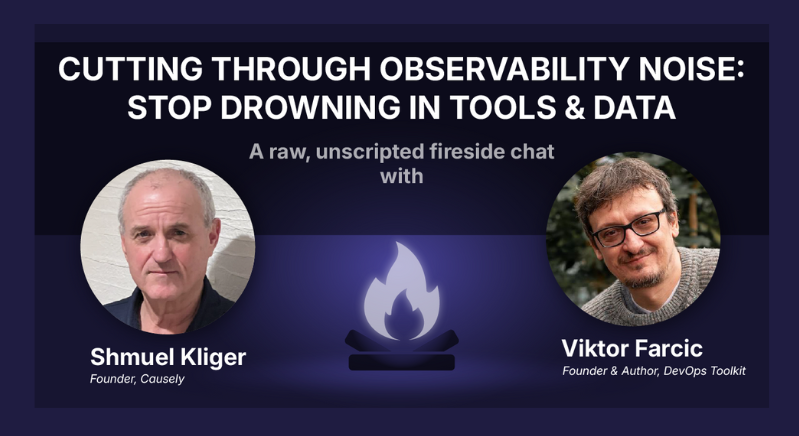
“You actually cannot do meaningful reasoning especially when it comes to root cause analysis with LLMs or machine learning alone. You need more than that.”
-Shmuel Kliger, Founder of Causely
Modern observability tools help ensure engineers have all the data they could hope for at their disposal. But these tools cause a certain amount of “observability overload”; these tools do not have an understanding of whether an observed anomaly is a real problem and, more importantly, they do not on their own accurately infer the why (i.e. root cause) behind the what (i.e. observed symptoms).
This was the focus of a recent conversation hosted by 10KMedia’s Adam LaGreca with guests Viktor Farcic (DevOps Toolkit) and Shmuel Kliger (founder of Causely). They explored why traditional observability approaches fall short on their own and what it will take to move beyond visibility toward real operational control.
The Signal Problem No One's Solving
The current state of reliability is defined by having too much data and not enough clarity. Alerts fire constantly and are often ignored. Dashboards contain all the data you could wish to have if you had all the time in the world to explore them and try to draw meaning from the data. What’s missing isn’t more data; it’s understanding. Without an understanding of cause and effect, teams are left guessing and leaning heavily on whichever domain experts they can grab at the moment.
Causal Reasoning as a Path Forward
The conversation centered on a new approach, causal reasoning, which leans away from bottom-up analysis of mountains of metrics, logs, and traces. By understanding service dependencies and identifying the cascading effect that load and code changes have on distributed systems, causal reasoning offers a way to eliminate the noise and focus on what truly matters for assuring reliable application performance. This helps reduce alert fatigue, speeds up troubleshooting, and frees engineers from the toil of reliability.
Redefining the Role of AI
While it makes logical sense to try to apply GenAI to the mountains of observability data we accumulate, leveraging LLMs without proper context will simply generate more noise at scale. By using causality to pinpoint the root cause of observed anomalies, a structured understanding of how systems behave can be used to get more efficient and effective outcomes from leveraging LLMs to automate operational work.
The Future of Reliability
Looking ahead, the vision is clear: fewer dashboards, fewer rabbit holes, and fewer hours lost to manual debugging. It’s a shift from observability to assurance. And for teams operating at the speed and scale of today’s distributed architectures, it’s a shift that can’t come soon enough.
🔍 Want to see this approach in action?
Check out the Causely sandbox or start a conversation with us: www.causely.ai